Hi, I'm Mike Suter-Tibble. I lead the team of digital performance analysts in DWP Digital. In the team we use a range of analytical tools to help digital teams iterate their services.
Google Analytics (GA) is one of the tools we use – it’s a web analytics service offered by Google that tracks and reports website traffic. It’s now the most widely used web analytics service on the Internet.
Developing a standard analytics implementation framework
As a team, we recently asked ourselves the question ‘What’s the best way to implement GA on the digital services we support?’
After a few years developing expertise using GA, we realised we needed to develop a standard implementation specification that could be used for all new services, with the least amount of modification required.
In turn we also needed to identify or develop the best way to tag different kinds of components on services (for example, radio buttons, validation errors, and outbound links).
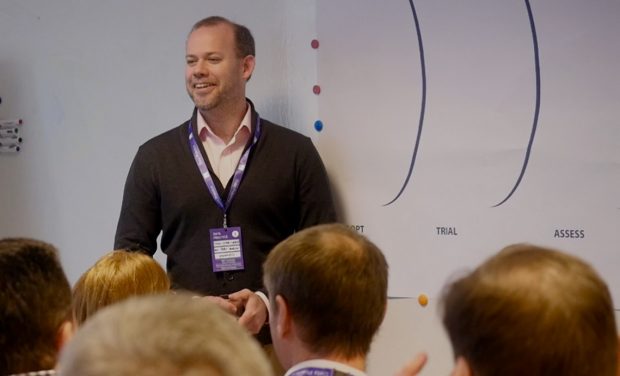
The aim was to reduce the amount of time we spend developing bespoke implementations, and ensure we have the data to answer questions when they come up.
After a lot of head scratching, workshops, and experiments with mocked up services, we’ve begun creating a standard approach to implementing GA, some examples of which I’d like to share.
Tracking the use of radio buttons to help improve question wording
A lot of DWP digital services enable people to apply for a benefit, loan, or grant so we need to ask users questions about their circumstances. Where only one response is possible to a question, ‘Yes’ or ‘No’, radio buttons are used. This makes them (probably) the most common component across DWP services.
As part of our work to develop the best standardised way to implement analytics on DWP services, we made a list of all the questions we’d been asked, and had asked ourselves about radio button questions. This included things like ‘what proportion of users answer ‘Yes?’, and ‘What proportion of users who answer ‘yes’ to this question answer ‘no’ to this one?’.
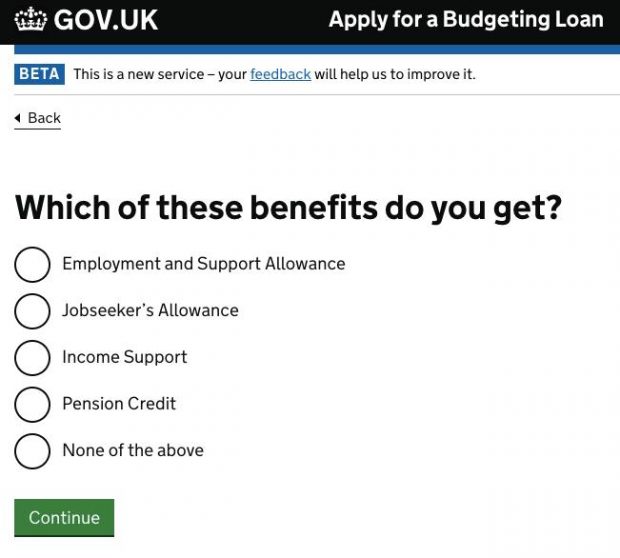
We’ve developed a way of tagging radio buttons that lets us answer the vast majority of questions we’ve been asked about these kinds of question. We can see the last answer a user selected, and create segments based on this; for example everyone who said someone was helping them complete their application lets us improve the assisted digital journey. We can also see how many users selected an answer, which helps us see which question might be causing drop-outs on pages with more than one question.
We can also see how often users select an answer then change that selection. Although we’d not been asked that question before, we thought it was one we should be answering. Users might change their answer because they found the question confusing; if this happens often, the question might need re-wording or splitting into multiple questions.
Implementing the ‘Multiple Answer Rate’ measure
Users might also change their answer on the basis of what happens next, for example if answering ‘yes’ to the question “do you file company accounts?” means users are told they need to post a copy of the latest accounts in, they might change their answer to ’no’. We developed a measure we’ve dubbed the ‘Multiple Answer Rate’, which is the number of sessions where the user answered a question, divided by the number of sessions in which the user selected more than one answer to that question.
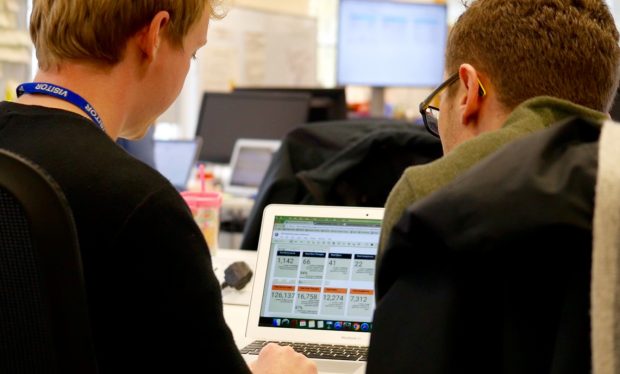
Tracking the ‘Multiple Answer Rate’, as we’ve called it, for every version of every question on every service will allow us to identify questions that might require some attention from Content Designers. It will also let us verify whether changes to question wording have made them easier for users to understand. Measuring it consistently across all services means we can get a sense of how similar but differently worded questions perform, and share learning across our services.
What’s next?
Ultimately, we’d like to be able to join all this up with data on which questions users most commonly give the incorrect answers to unintentionally (i.e. error, rather than fraud). This would let us identify the questions that aren’t achieving their main aim, which is to gather accurate information from users. At the moment, that’s still a work in progress.
I’m also going to write further blog posts about linking online activity to outcomes and using the Median rather than the Mean for time metrics. Look out for those!